Scientists Develop New Algorithm That May Provide Insights Into Battery Corrosion
Argonne researchers have created an automatic technique that can fill in gaps in X-ray data.
Putting together a jigsaw puzzle is a great activity for a rainy Sunday afternoon. But the somewhat more difficult process of quickly assembling 3D scientific jigsaw puzzles — atomic structures of different materials — has recently gotten a lot easier, thanks to new research that pairs high-powered X-ray beams with advanced computing methodologies.
Researchers at the U.S. Department of Energy’s (DOE) Argonne National Laboratory have developed a new technique that accelerates the solving of material structures from patterns uncovered in X-ray experiments. The technique allows researchers to study certain properties, such as corrosion or battery charging and discharging, in real time.
“With the kind of diffraction we’re doing, getting the phase information is a challenge; it’s like figuring out how all the pieces (of the jigsaw) fit together solely based on the colors you can see on each piece.” — Yudong Yao, Argonne National Laboratory.
The technique, called AutoPhaseNN, is based on a method called machine learning, which trains an algorithm on certain experimental data and then uses it to choose the most likely outcome of the current experiment. The data used in this case are created by shining ultrabright X-ray beams from Argonne’s Advanced Photon Source (APS) on a material and capturing the light as they bounce off, a process called diffraction. The APS is a DOE Office of Science user facility at Argonne.
New techniques are important as the APS is in the midst of a massive upgrade, which will increase the brightness of its X-ray beams by up to 500 times. This means that more data will be gathered more quickly once the upgraded APS comes online in 2024, and scientists will need a way to keep up with analysis of that data. Machine learning solutions such as AutoPhaseNN will be a vital part of the more rapid data analyses needed in the future at APS, as well as similar facilities around the globe.
AutoPhaseNN is an example of an “unsupervised” machine learning, which means that the computer algorithm learns from its own experience how to do a computation more accurately and efficiently, without having to be trained with labeled solutions that have already been figured out, a process which usually involves human intervention.
“This new algorithm is essentially able to solve what we call an inverse problem, going from the pieces of the puzzle to create the puzzle itself,” said Argonne computational scientist and group leader Mathew Cherukara, an author of the study. “In essence, we’re taking a set of observations and trying to identify the conditions that created them. Instead of solving the puzzle by iterating the process of trial and revision based on the prior knowledge, our algorithm assembles the puzzle from the broken pieces in a single step.”
Getting information about the structure of a material requires scientists to obtain information pertaining not only to the amplitude of the diffracted signal, but also its phase. However, the amplitude, or intensity, is the only part that can be directly measured.
Because the X-ray beams used to illuminate the sample are coherent — meaning they all share the same phase initially — whatever change to the phase occurs as a result of the diffraction can be mapped back to the sample itself, said Argonne nanoscientist and co-author Henry Chan.
“Phase retrieval is essential for understanding the structure — most of the relevant information is found in the phase,” said lead author Yudong Yao, an Argonne X-ray physicist at the time of this research. “With the kind of diffraction we’re doing, getting the phase information is a challenge; it’s like figuring out how all the pieces fit together solely based on the colors you can see on each piece.”
For conventional, supervised neural networks to solve this inverse problem, the researchers would have had to pair “broken puzzles” with fully assembled examples so that the neural network could have something to train against. With an unsupervised neural network, the algorithm can learn to stitch together the puzzle from just the broken pieces. The resulting network is fast, accurate and (unlike conventional methods) capable of providing 3D images in real time to scientific users of facilities like the APS.
A paper based on the study, “AutoPhaseNN: unsupervised physics-aware deep learning of 3D nanoscale Bragg coherent diffraction imaging,” appeared in the June 3 online edition of NPJ Computational Materials. In addition to Cherukara and Yao, other authors include Argonne’s Henry Chan, Subramanian Sankaranarayanan, Prasanna Balaprakash and Ross Harder.
The work was funded by DOE’S Office of Science (Office of Basic Energy Sciences). This research used resources of the Center for Nanoscale Materials and Argonne Leadership Computing Facility, which are DOE Office of Science user facilities, and the Laboratory Computing Resource Center at Argonne.
About the Advanced Photon Source: The U. S. Department of Energy Office of Science’s Advanced Photon Source (APS) at Argonne National Laboratory is one of the world’s most productive X-ray light source facilities. The APS provides high-brightness X-ray beams to a diverse community of researchers in materials science, chemistry, condensed matter physics, the life and environmental sciences, and applied research. These X-rays are ideally suited for explorations of materials and biological structures; elemental distribution; chemical, magnetic, electronic states; and a wide range of technologically important engineering systems from batteries to fuel injector sprays, all of which are the foundations of our nation’s economic, technological, and physical well-being. Each year, more than 5,000 researchers use the APS to produce over 2,000 publications detailing impactful discoveries, and solve more vital biological protein structures than users of any other X-ray light source research facility. APS scientists and engineers innovate technology that is at the heart of advancing accelerator and light-source operations. This includes the insertion devices that produce extreme-brightness X-rays prized by researchers, lenses that focus the X-rays down to a few nanometers, instrumentation that maximizes the way the X-rays interact with samples being studied, and software that gathers and manages the massive quantity of data resulting from discovery research at the APS.
This research used resources of the Advanced Photon Source, a U.S. DOE Office of Science User Facility operated for the DOE Office of Science by Argonne National Laboratory under Contract No. DE-AC02-06CH11357.
Argonne National Laboratory seeks solutions to pressing national problems in science and technology. The nation’s first national laboratory, Argonne conducts leading-edge basic and applied scientific research in virtually every scientific discipline. Argonne researchers work closely with researchers from hundreds of companies, universities, and federal, state and municipal agencies to help them solve their specific problems, advance America’s scientific leadership and prepare the nation for a better future. With employees from more than 60 nations, Argonne is managed by UChicago Argonne, LLC for the U.S. Department of Energy’s Office of Science.
The U.S. Department of Energy’s Office of Science is the single largest supporter of basic research in the physical sciences in the United States and is working to address some of the most pressing challenges of our time. For more information, visit https://energy.gov/science.
By Jared Sagoff. Courtesy of Argonne National Laboratory.
Have a tip for CleanTechnica? Want to advertise? Want to suggest a guest for our CleanTech Talk podcast? Contact us here.
Latest CleanTechnica.TV Video
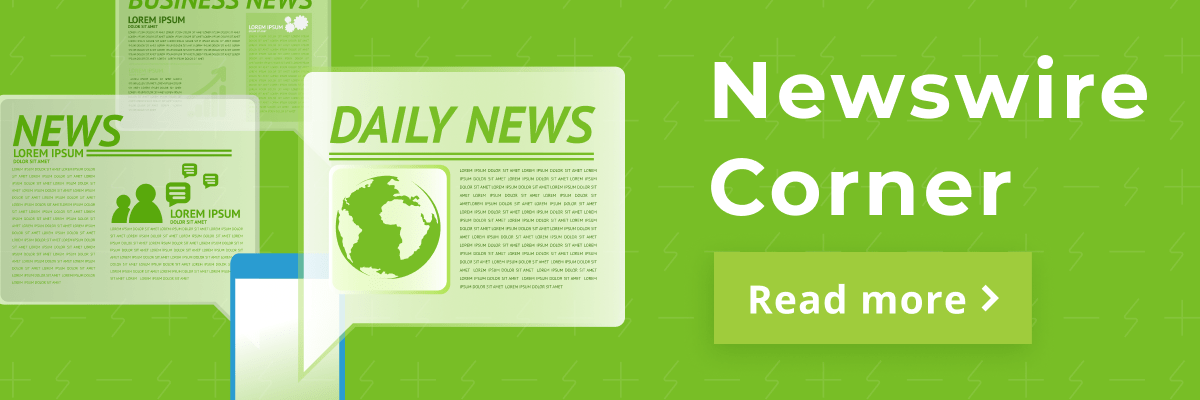
CleanTechnica uses affiliate links. See our policy here.