The Water Knife Meets Neuromancer: Machine Learning For Southwestern US Water Quality
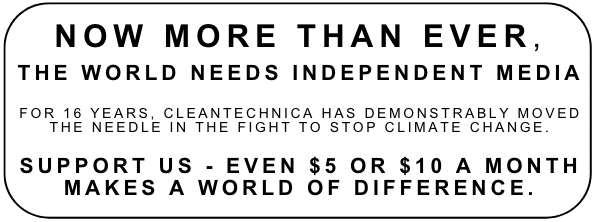
In the climate-fiction dystopia The Water Knife, by Paolo Bacigalupi, the American southwest is in the middle of a near-future, post-climate change dissolution. I provided a less fictional and more balanced view of the region in a recent CleanTechnica article, After Florida, Arizona’s Real Estate Market Will Be Hit Next By Global Warming. And in William Gibson’s dystopian cyberpunk novel Neuromancer, an artificial intelligence starts messing with human lives in order to achieve its own ends. How do these threads possibly intersect?

That’s where a recent joint announcement from Ketos and the Southern Nevada Water Authority comes in.
Thankfully, while the Authority is working with Ketos, a company with machine learning components, we aren’t at the point of Skynet for our water supply yet. But Ketos is starting take advantage of the opportunities that advances in artificial intelligence provide to improve water usage and the quality of outcomes of systems where water is a key input or output.
As may be obvious from the logo, Ketos is a phonetic derivation of cetos, the Greek word for any huge sea monster, but one they assert means orca or killer whale, the fast-moving, highly intelligent mammalian predator. Their focus on orcas is as visible, romantic, apex predators that are threatened by our mistreatment of our common water resource, to help keep in mind that being good stewards of our water is necessary.
Ketos has two products, both of which share many components and design principles. The first, the Ketos Wave, is a water flow measurement tool, and the second, Ketos Shield, is a water quality measurement tool.
They aren’t unique in the industry, but they do have some interesting and differentiating features. The Wave is designed to be standalone, self-powered monitor as one of many such monitors. The Wave uses the water flowing through it to generate electricity to run its sensors and communication. They’ve built in a proprietary mesh network that allows it to communicate in the absence of existing wifi and cellular signals, with the data hopping across the Wave units until it has a connection point to the larger electronic world.

The design point is for places where water is flowing, and communications don’t exist but nearer-field electronic communication is possible without wiring or external routers. The communication stack is based on a LoRaWan, a common long range, low power wireless chipset that is used in a lot of Internet of Things (IoT) networks worldwide.
The Shield, on the other hand, is designed for places where communication and power exists, more typically points of usage and entrance to facilities. It uses both optical spectrographic and other proprietary sensors to provide a broad range and quality of data about the water, including detection of heavy metal toxins such as lead, copper, arsenic, chromium, and the like. Communication is through Ethernet or cellular connections.
They’ve built both on a common, two-way communication fabric, but so far, so standard. IoT devices with sensors and communications aren’t a dime a dozen, but they are much more pervasive than they were a decade ago. Where it starts to get more deeply interesting is in the use of machine learning components.
Machine learning is an area of substantial advancement over the past few years. A standard image library, ImageNet, for training image processing systems has emerged. A layered architecture for visual image processing has emerged, with the three primary researchers, Yoshua Bengio, Geoffrey Hinton, and Yann LeCun, two Canadians and an American who split their time variously between universities, Facebook and Google, sharing the million dollar Turing award for their innovation and insights. RetinaNet, as an example, is a pre-baked, multi-layer image recognition neural net that starts with the ImageNet image set and finds and assembles patterns of greater and greater abstraction, which can be included in new solutions with limited new sets of images to find value without having to recreate all the primitive learning. One up-to-date, freely available, machine-learning course uses RetinaNet to correctly identify cat and dog breeds with 96% accuracy in two minutes of Amazon Web Services training on a small number of cat and dog pictures. On the language processing side, a similar reusable neural net called ELMo has provided a step-change in natural language processing and translation with deep, contextualized meaning sets.
Fair warning for regular CleanTechnica readers. Just as last year I went broad and deep on blockchain for cleantech solutions, this is one of the early articles in a series on the application of machine learning to our transformation into the low-carbon future.
One of my primary contacts into the machine learning space is my on-and-off collaborator David Clement. He and I worked together on a few Vancouver, BC, dot-com IPO development projects and had side projects exploring everything from swarm-based robotics — insights from that effort informed my article on Tesla’s advantages in their approach to autonomous cars, Tesla Has The Right Approach To Self-Driving Cars — to industrial design. He’s spent the past two years going deep and broad as a hands-on architect in machine learning technologies, and has created real-time video processing capabilities that are at the cutting edge of what is possible. Imagine near movie quality motion-capture from iPhone videos of people doing anything while wearing normal clothes, and you’ll start to understand the domain he’s innovating in.
He and I are exploring the world of machine augmented human attention systems, and of course one of my focus areas is clean technology, so the overlap and Ketos’ positioning to attract my attention is obvious.
Unpacking that, neural nets can take in any stream of regularly updated, complex data, and as long as it can be turned into images or text, it’s possible to rapidly find features of interest in the data and start using machine learning components in a solution to deliver value. The machine learning component keeps paying attention and auto-detects things it can observe easily, humans can figure out which of those features have meaning, and in many cases humans can also train it to relate features that the machine can’t easily see itself, allowing even greater insights to emerge. Imagine a human expert seeing two peaks in a spectrographic analysis which they know are meaningful, drawing a linkage between the apparently disparate features and then having the neural net automatically detect them going forward.
One of the core concepts is ongoing attention to a data stream or streams of relevance to a problem domain. The second is that a relatively low-flexibility neural net with effectively infinite ability to pay attention to the streams exists in machine learning tools. The third is that a high-flexibility neural net exists in humans who can work with and train the computerized neural net to see new things, which it will just be able to keep seeing. The human and computerized neural nets collaborate in paying attention better and better.
Tying this back to Ketos, every new customer that they install the Wave and Shield sensors for is feeding their neural net, which keeps getting better at seeing things in the sensor feeds, things which can be of value to all their customers through their central portal. Just as Tesla early adopters noticed that their cars got better at driving very rapidly after Autopilot was turned on in certain circumstances, something that was due to Tesla’s machine learning system seeing human corrections in certain circumstances and hence training itself to be better, so every Ketos customer will see improvements as more customers come into the system.
But that’s not all. The extension point for this is beyond just water flow and quality. The company can extend the feeds with customer specific data and start seeing features and optimizing outcomes for the larger system. The neural net learning system is extensible to the domains where the water is used, in other words, and customers could start seeing optimization beyond just water usage. Look back at the diagram of where Ketos sensors might be installed in agriculture and industry, and start thinking of other sensor sets that include outcome data for crops or quality measures, and the insights that could be gained to improve processes and results.
The Southern Nevada Water Authority is focused on provision of the highest quality of water to its customers, so it’s less focused on changing processes. However, they believe that they should be able to substantially reduce the cost of water quality testing across 20 contaminants. With the high likelihood of fresh water continuing to become rarer and hence more expensive in that part of the world, finding other efficiencies to reduce cost increases is critical.
The work of Ketos and the Authority continue to push back the dystopian future of The Water Knife so that Las Vegas gamblers and wedding parties will still be able to drink the water for years or decades to come. And Ketos is working to create a vastly less intelligent but much more helpful neural net than either the Wintermute or Neuromancer AIs of Gibson’s eponymous book.

Sign up for CleanTechnica's Weekly Substack for Zach and Scott's in-depth analyses and high level summaries, sign up for our daily newsletter, and follow us on Google News!
Whether you have solar power or not, please complete our latest solar power survey.
Have a tip for CleanTechnica? Want to advertise? Want to suggest a guest for our CleanTech Talk podcast? Contact us here.
Sign up for our daily newsletter for 15 new cleantech stories a day. Or sign up for our weekly one on top stories of the week if daily is too frequent.
CleanTechnica uses affiliate links. See our policy here.
CleanTechnica's Comment Policy